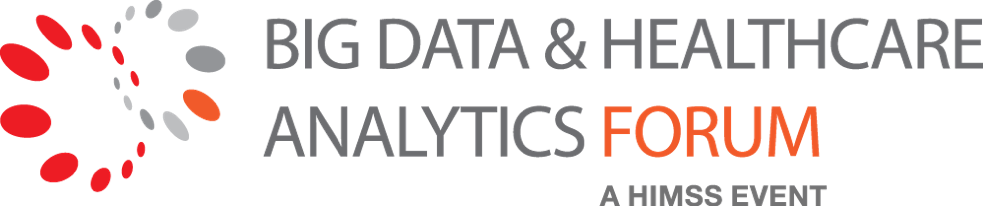
A futuristic look at the next ten years of healthcare delivery, review of the current state of AI/ML initiatives, presentation of real success stories associated with use of ML-driven analytics from several IDNs and provider practices – all these were the main subjects of discussion at Big Data and Healthcare Analytics Forum in Boston (October 22nd-23rd ,2018).
Michael Rogers opened the first day by sharing his vision of the next decade. According to him, we will be seeing massive vertical consolidations of vendors and an imminent advent of low-cost delivery of health and wellness services by Amazon Health or CVS Wellness. There will be smart sensors everywhere. The IoT powered by AI will ensure seamless handoff of data – home, car, and hospital. Maturing digital standards will help with interoperability. Patient diagnostics and predictive analysis of outcomes will be fueled by use of Genomic and Germline data. Customer service will also be improved by using AI. At the same time, some of the downsides include de-scaling of the human factor and loss of social skills among the youth population.
These predictions leave all of us in the industry with the thoughts on where to distribute the engineering efforts and how to organize technology roadmaps to better address the operational and analytical needs of caregivers. With the influx of massive amounts of incoming data, we all need to be prepared to house and process it. With an imminent advent of Amazon-like consumer-oriented health offering, the interoperability demands will rise exponentially.
An overarching consensus among the participants is that while today Healthcare Analytics powered by AI/ML have already gained technological and operational maturity, the existing capabilities are still not fully addressing the needs of clinicians. Today the healthcare industry is not well equipped to implement transformation of vast and complex raw data into actionable knowledge. The feedback from hospital executives and physicians says that in many cases they are repeatedly told by the analytical and reporting data what they already know. Readily available data is descriptive, but not necessarily prescriptive, which is what really needed. There are numerous implementation success stories presented by IDNs, AI/ML vendors and ACOs, but most of them are isolated efforts, focused on specific areas and outcomes.
In order to build up on the existing achievements or start from inception, hospitals first need to develop a strategic organizational plan. As part of the plan, several key considerations have to be made and the key answers have to be identified and socialized internally and with the 3rd parties. Â Some of these considerations should include:
- Identify consumers of the analytical offerings and data literacy of target recipients of data
Start the program by identifying the users/consumers of analytical offerings in different departments. Understand the existing needs and identify the gaps and opportunities for AI to add value. While working with the future users of the offerings, consider their levels of data literacy. We often do not recognize the value of data literacy and importance of training. Having a well-polished report delivered to a physician or a nurse is not enough. The users need to be trained on how to interpret and navigate the clinical and operational data delivered to them. Workforce development is especially valuable when instead of traditional reports users are offered complex analytical front-ends with “what-if†modeling capabilities. Consider future investments into training programs, including possible train-the-trainer initiatives. - Define the Operational and Clinical Objectives
Work with your business users and clinical leadership on clearly defining the objectives of the analytics. Avoid the common pitfall of first building the offering and then looking for applicability of the results in the organization. This needs to be a collaborative, cross-functional consensus-driven effort. Lack of internal alignment will result in slow adoption and potentially in refusal to accept the data. In today’s clinical practice world, physicians are experiencing the overload of incoming data to be processed. Instead they need targeted, actionable sets of knowledge they could use to treat patients. - Identify your staffing needs
Depending on the long-term objectives, your organization may decide to hire staff data scientists and engineers. Alternatively, numerous software vendors, both onshore and offshore are offering their services in helping organization bootstrap their Big Data initiatives. When deciding on a staffing strategy, consider availability of required skills on the local market, existing commitments, deadlines, ownership of IP and training data, potential concerns with ePHI - Establish and Strengthen Data Governance as an enterprise function
Importance of Data Governance as a function needs to be recognized organizationally, including early data identification, classification, and designation of organizational roles as it pertains to use of data, such as data custodians and producers. Consider sources of data, collection mechanisms, internal vs externally gathered, and legal aspects of 3rd party data use. In order to collect and house some of the required data, changes need to be made to the existing operational systems, such as EHRs. One good example is social and behavioral determinants. They have significant predictive power, but typically not available in traditional EHRs. Another consideration is portability of data. The collected data may be liquid, but not necessarily portable to other systems or healthcare constituencies. Another important consideration is data privacy and security - Review and incorporate use of the regulatory compliance (FDA) frameworks
Scott Gottlieb, MD, FDA Commissioner, speaking at AcademyHealth 2018 event on April 26 revealed a new FDA framework and software validation tools, which clearly indicates FDA’s recognition of Artificial Intelligence tools and technologies as one of the prime subjects of interests. For vendors and consumers of AI/ML technologies, early adoption of the FDA framework may accelerate the production cycle. - Determine longevity and lifecycle of predictive models
Statistical models used for risk-adjustment prediction of outcomes require periodic re-calibration as the training data changes due to a variety of socioeconomic, demographic and other factors. For example, merger with another regional IDN or a hospital may result in availability of new data. The frequency of re-calibration needs to be determined and included into operational plans. - Define your cloud strategy
Working with Big Data requires extensive hardware and software elastic resources. Deciding on whether to use a private, commercial or a hybrid cloud is a going to shape the development and operational plans. Today we have several mature vendors who offer HIPAA-enabled secure and elastic environment with burst-on-demand capabilities and pre-configured analytical/BI tools. The decision process should factor the existing enterprise agreements, standards, skill sets of data scientists and developers.
- Recognize the importance of AI as a social function
In the underdeveloped countries where access to physician specialists is limited or even practically impossible for certain groups, use of Artificial Intelligence for diagnostic/medical imaging could turn out to be a real life-saver. With a limited availability of qualified dermatologists, for instance, one of the use cases discussed at the conference was early identification of Melanoma. Artificial Intelligence is definitely not meant to be a substitute for a physician, as it was discussed in the presentation by Dr. John Halamka, MD, but it could serve as a tool of Augmented Intelligence, reducing the well-recognized physician burnout and making healthcare more accessible worldwide.
Facebook Comments